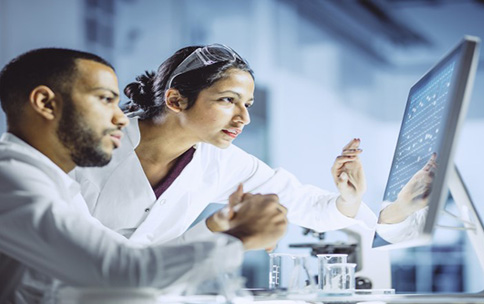
In 2023, approximately 1.9 million Americans will be diagnosed with cancer. There are many options for treating cancer, including surgery, radiation therapy, chemotherapy, and targeted therapy. Unlike chemotherapy, which kills cells that grow and divide rapidly (including healthy cells), drugs used for targeted cancer therapy target proteins that affect the growth and spread of cancer cells. Though the use of targeted therapies has led to improved outcomes for several types of cancer, the ability to predict how a cancer might respond to a targeted treatment is lacking, in part because prediction models do not adequately account for all of the molecular consequences that result from treating a target with a drug. This prompted researchers from the Common Fund’s Illuminating the Druggable Genome (IDG) program to investigate an approach to improve the prediction of cancer response to targeted therapy.
A primary goal of the IDG program is to discover information about proteins that are not well-studied among three protein families. One protein family the program studies is kinases, which are proteins that function in many cellular processes and, if improperly regulated, can lead to cancer. Because of their role in cancer, kinases are often a focus for targeted drug development. Kinases, however, do not function in isolation. Instead, they interact with each other in a complex network called the kinome. Research suggests that targeting a kinase with a drug can result in subsequent changes that might impact the entire kinome. This interactive nature of the kinome makes it difficult to predict how an instance of cancer may respond to targeted kinase therapy.
To address this challenge, Dr. Shawn Gomez and colleagues developed an approach to predict when targeting specific kinases would impact cell viability, a measure of cell survival after treatment with a drug. First, the researchers integrated information on gene activity, protein function, the kinome, and cell viability from cancer cell line datasets. Then the researchers used machine learning, a type of artificial intelligence where computer algorithms use data to make predictions, to predict the response of cancer cell lines when targeting specific kinases. The machine learning models that included information on gene activity and the kinome were able to predict cell viability with a high degree of accuracy. This work suggests that incorporating information on the kinome may facilitate the development of effective targeted therapies for cancer.
Reference:
Kinome inhibition states and multiomics data enable prediction of cell viability in diverse cancer types. M.E. Berginski, CU Joisa, B.T. Golitz, S.M. Gomez. PLoS Comput Biol. 2023 Feb 21; 19(2):epub.