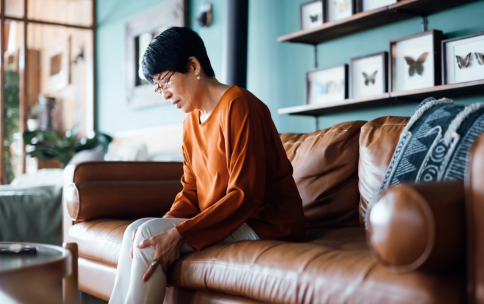
Preventing chronic pain following an acute pain event, such as an injury or surgery, poses a significant challenge. Chronic pain, which lasts beyond the normal healing period, can significantly decrease quality of life, and disrupt many aspects of daily living. To understand the causes and risk factors for developing chronic pain, more accurate methods of measuring pain are required
In a new study in the journal PAIN, researchers from the NIH Common Fund Acute to Chronic Pain Signatures (A2CPS) program use statistical modeling and Ecological Momentary Assessment (EMA) to examine the best ways to measure pain. EMA is an important tool in pain research that provides detailed information on the frequency and intensity of pain by collecting data multiple times a day. Pain is commonly measured on a scale of 0 to 10; however, there is no universal method for defining chronic pain using EMA. The research initiatives of A2CPS aim to support the development of biomarkers, or biological indicators, to predict if chronic pain will develop or resolve after acute pain events. The hope is that multiple biomarkers could be combined to provide predictive “signatures” that could expedite therapy development and inform strategies for chronic pain prevention.
Pain scores can vary widely within and between individuals, affecting the accuracy of traditional statistical models. This study introduces a new model that considers the relationship between pain variability and average pain scores. The researchers use statistical modeling to examine the best ways to measure pain and determine if it should be classified as chronic. By defining chronic pain as a score of at least a 3 on a scale of 0 to 10, the researchers found that simplifying the categorization by separating the pain scores into two groups (“less than 3” and “3 or more”) reduced the ability to detect true differences in pain scores. However, by using the full pain scale (0 to 10), they detected smaller differences in pain levels more accurately. This approach of categorizing pain, using a continuous scale rather than a set of binary groups, increases sensitivity, meaning that this approach is better at accurately classifying chronic pain. The researchers noted that the new model for pain score variability might not be optimal for all populations, and that alternative models might offer better predictions for specific groups. In addition, the work suggests that simple methods for estimating a patient’s average pain score are sufficiently accurate, and that converting average pain intensity data into a binary yes-or-no answer of chronic pain should be avoided.
Read more about chronic pain research and the A2CPS program.
Reference:
Leroux A, Crainiceanu C, Zeger S, Taub M, Ansari B, Wager TD, Bayman E, Coffey C, Langefeld C, McCarthy R, Tsodikov A, Brummet C, Clauw DJ, Edwards RR, Lindquist MA, A2CPS Consortium. Statistical modeling of acute and chronic pain patient-reported outcomes obtained from ecological momentary assessment. PAIN. 2024 May 7;10.1097/j.pain. 0000000000003214. doi: 10.1097/j.pain.0000000000003214