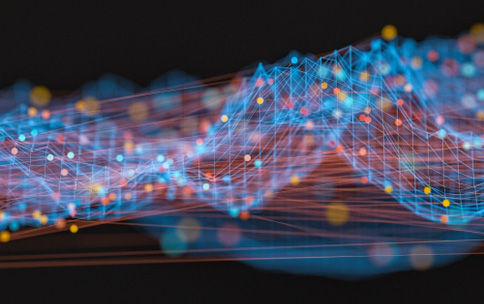
Researchers from the NIH Common Fund Knockout Mouse Phenotyping Program (KOMP2) are generating a massive amount of useful data from thousands of mice. KOMP2 is part of the International Mouse Phenotyping Consortium (IMPC) (link is external) effort to generate "knockout mice" for every protein coding gene in the mouse genome – which then carries out a range of tests to understand each gene’s biological function.
Experimental data from the knockout mice must be carefully compared to control data from normal mice and then appropriately analyzed to be meaningful. By nature of the high-throughput, large-scale study design, more control data are generated over time than data from each unique knockout group tested. While these ever-growing control data can help make analyses more powerful, they can also add complications because of larger variation over time with “batch” effects. Batch effects are unintended influences of variables like seasons, different personnel performing tests, and different reagent lots that can affect data. To account for this unintended variability, KOMP2 researchers developed a “soft windowing” method designed to select a time window that would include the best control data to use. The approach uses an adaptive window, meaning data from control mice measured most concurrently to the knockouts are given the strongest weight of all the control data, while data collected earlier or later had less weight. When validating their soft windowing approach, KOMP2 researchers found that the rate of false positive discovery went down. A false positive result is one that is unlikely to be biologically meaningful and most likely happened by chance. By lowering this sampling “noise,” the researchers were able to establish more associations between genes and function than with traditional methods and therefore to provide a clearer picture of the biological function of many more genes. The method is freely available in the R package SmoothWin (link is external)and is intended to be generalizable and benefit large-scale human projects like the UK Biobank (link is external)and All of Us.
Reference:
Soft Windowing Application to Improve Analysis of High-throughput Phenotyping Data. Haselimashhadi, H., J. C. Mason, V. Munoz-Fuentes, F. Lopez-Gomez, K. Babalola, E. F. Acar, V. Kumar, J. White, A. M. Flenniken, R. King, E. Straiton, J. R. Seavitt, A. Gaspero, A. Garza, A. E. Christianson, C. W. Hsu, C. L. Reynolds, D. G. Lanza, I. Lorenzo, J. R. Green, J. J. Gallegos, R. Bohat, R. C. Samaco, S. Veeraragavan, J. K. Kim, G. Miller, H. Fuchs, L. Garrett, L. Becker, Y. K. Kang, D. Clary, S. Y. Cho, M. Tamura, N. Tanaka, K. D. Soo, A. Bezginov, G. B. About, M. F. Champy, L. Vasseur, S. Leblanc, H. Meziane, M. Selloum, P. T. Reilly, N. Spielmann, H. Maier, V. Gailus-Durner, T. Sorg, M. Hiroshi, O. Yuichi, J. D. Heaney, M. E. Dickinson, W. Wolfgang, G. P. Tocchini-Valentini, K. C. K. Lloyd, C. McKerlie, J. K. Seong, H. Yann, M. H. de Angelis, S. D. M. Brown, D. Smedley, P. Flicek, A. M. Mallon, H. Parkinson and T. F. Meehan 2019 Oct 8;btz744. doi: 10.1093/bioinformatics/btz744. [Epub ahead of print]. PMID: 31591642.